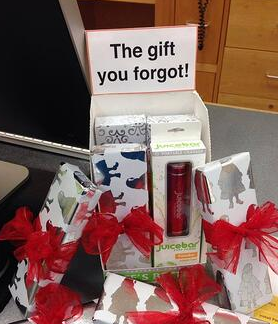
We have not experienced a global pandemic like the coronavirus in last 100 years. The sheer increase in demand for everyday necessities like toilet paper, sanitary wipes and bottled water is putting undo stress on a lean global supply chain.
It is testing the agility of many retailers and consumer packaged goods (CPG) companies as they attempt to ramp up manufacturing facilities and logistical operations while struggling to keep up with consumer demand.
Business executives are looking to data, analytics and technology for answers on how to predict and plan for the surge and, ultimately, the decline in consumer demand. It is significantly easier to shut down facilities than it is to quickly boost production and capacity.
The biggest unknown is whether there will be a delayed economic recovery or a prolonged contraction. Regardless of the outcome, retailers and their CPG suppliers will need to think ahead and be prepared to act quickly.
How to predict and plan for the surge and decline in consumer demand patterns
Retailers and their CPG suppliers are the backbone of the consumer goods supply chain and a lifeline to their customers. Their ability to operate efficiently is determined by the weakest link in the end-to-end supply chain.
The current crisis has changed the make-up of the average grocery basket making it difficult to predict rapidly changing demand patterns. As a result, the current supply chain is struggling to keep up. Restoring balance will require changes in the way demand forecasting and planning are conducted by both retailers and CPG companies.
Navigating the current climate will require new intelligence, resilience and more dependence on advanced analytics and machine learning than ever. Here are six actions that can improve retailers’ and consumer goods suppliers’ ability to predict the changing demand patterns.
1. Use downstream data that reflects true consumer demand
First and foremost, analyze and forecast the POS data collected through store scanners. Use it to determine the shift in demand patterns for your products in order to more accurately forecast the mix within the average market basket.
It is even more important to focus on forecasting the lower product mix as it will indicate which items have the highest demand velocity as well as those products with the lowest demand. Most CPG companies receive POS data directly from their retail customers on a daily and/or a weekly basis and POS data is the truest consumer demand signal.
Several CPG companies have been analyzing and forecasting the POS data for products sold to their top 20 global grocery retail customers, are recognizing significant shifts in consumer demand patterns almost immediately and are acting accordingly. They have also detected the increase in demand for their products on Amazon.com as consumers shifted from brick and mortar stores to online purchases during this same period.
Using POS demand history and revised future forecasts as a leading indicator in their shipment models, CPG companies can more accurately predict supply replenishment to those same grocery retail customers.
This new consumption-based forecasting approach using the MTCA (multi-tiered causal analysis) process has allowed CPG companies to significantly improve not only shipment forecasts but to also detect turning points in demand patterns much faster than traditional shipment models allow. (See Chase, 2016, Next Generation Demand Management: People, Process, Analytics and Technology, John Wiley).
2. Adopt and implement advanced analytics and mL algorithms in your demand forecasting & planning
Implementing advanced analytics and machine learning algorithms can help spot abnormalities quickly and adjust immediately. Several SAS customers who have recently implemented advanced analytics and machine learning technology were able to predict the shifts in demand patterns quickly, while their legacy systems were failing to predict those changes.
Customer Example: Recently, we had a discussion with a large French retailer’s data scientist about how the brand was coping with the COVID-19 crisis, and how their new SAS solution (designed to forecast warehouse shipments) was handling the shift in demand patterns. The data scientist explained that the new forecasting was performing very well while their legacy solution was crashing.
This comparison illustrates that even when the shifts in demand patterns were significant, the autotuning advanced analytics models quickly adapted.
3. Implement a short-term demand forecasting and planning process
Implementing a short-term (one to eight weeks) forecast that utilizes advanced analytics and machine learning to predict weekly and daily demand using sales orders and shipments in combination with POS data.
Using POS data as a leading indicator in the models (along with sales promotions and events) allows a retailer to calculate not only the promotion lifts but the shifts (anomalies) in short-term demand patterns.